Department of Engineering
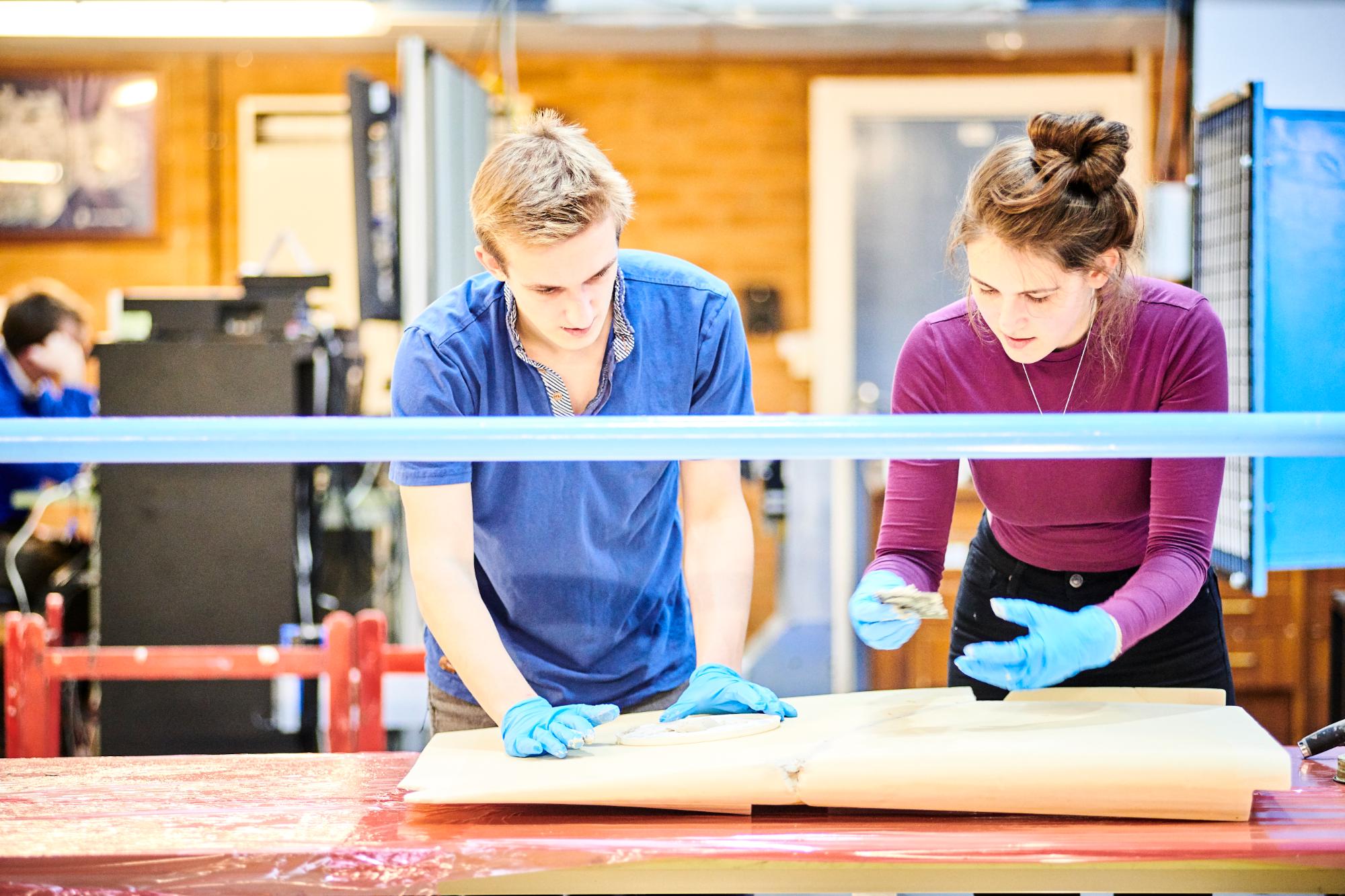
Welcome to Engineering at Durham
As part of the Russell Group of leading research-intensive universities, Durham University has a longstanding and powerful commitment to research and research-led teaching. We are recognised as one of the leading centres of research in Engineering in the world. Our research covers a wide range of topics, and we encourage interdisciplinary research within the Department as well as national and international cooperation.
Our degree programmes are accredited by the Professional Engineering Institutions on behalf of the Engineering Council. This means that graduating students will be deemed to have satisfied certain academic requirements. The MEng programmes fully satisfy the educational base for a Chartered Engineer (CEng). The BEng programmes partially satisfy the educational base for a Chartered Engineer (CEng), and will fully meet the requirement when complemented with accredited Further Learning. The accredited MSc programmes meet the accreditation requirements of the Engineering Accreditation Board (EAB) for Further Learning for a Chartered Engineer (CEng) for candidates who have already acquired an accredited CEng (partial) BEng (Hons) or an accredited IEng (Full) BEng/BSc (Hons) undergraduate first degree.
Look Closer at Department of Engineering
Take a lightning tour of day-to-day life at the Department of Engineering with one-minute video, filmed on and around campus.
Study with us
Undergraduate study
We have outstanding course content that equips young engineers with the problem solving skills of the 21st century.
Postgraduate study
We have a rich research background, which can be a huge advantage to any prospective postgraduate student in our fields of expertise.
Our research
This is a very interesting and exciting time to become an Engineer and Durham University provides the perfect environment to begin this journey.
Find out more
What's new?
BGA Masters Dissertation Prize 2024 Awarded to MEng Graduate Michael Dixon
We are delighted to announce that Michael Dixon, an MEng graduate, has been awarded the 2024 British Geotechnical Association Masters Dissertation Prize.
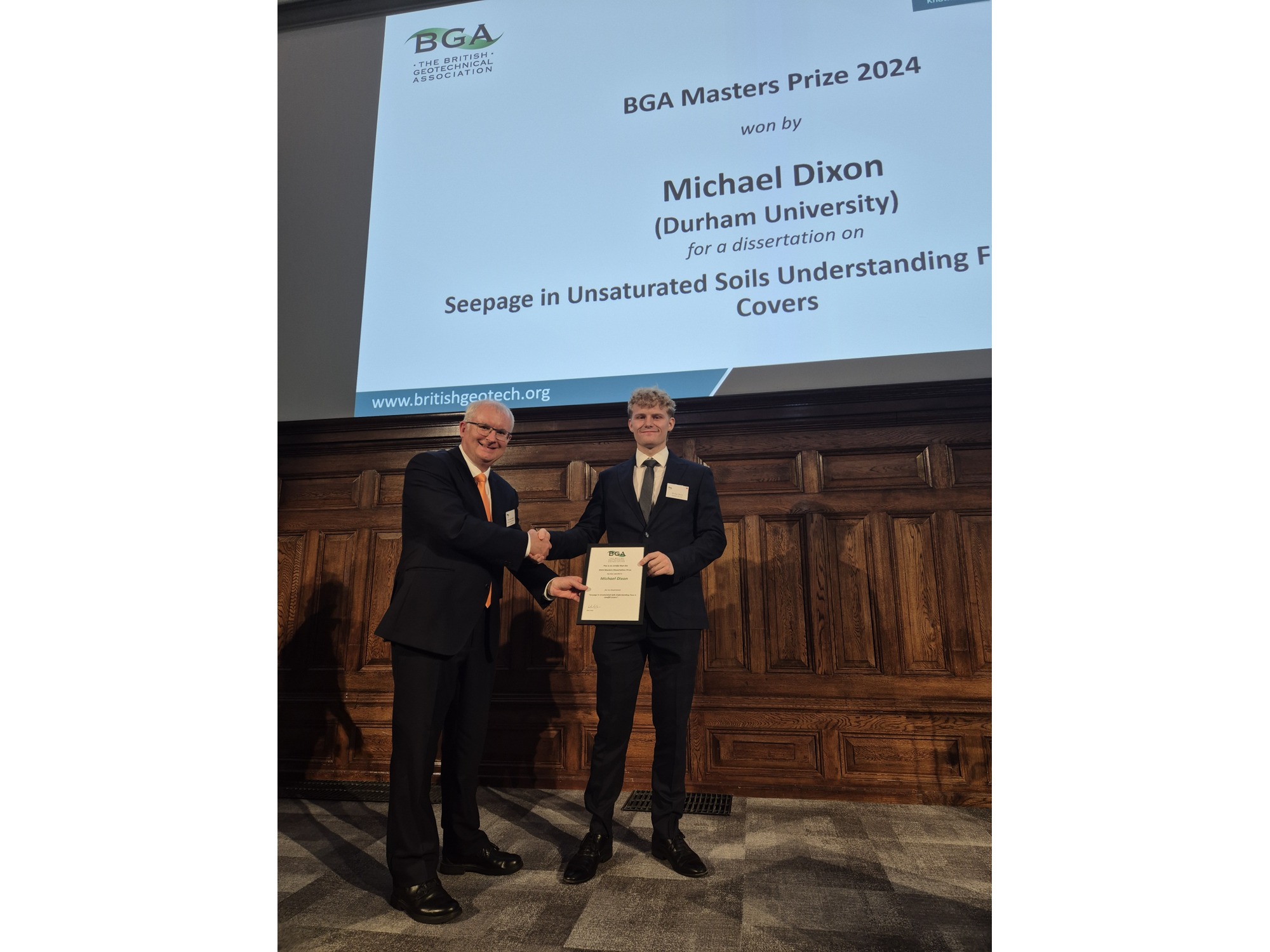
Engineering PhD Student Presents at Parliament in STEM for Britain Finals
£11.5m project to turn sewage into sustainable fuels
Find us on social media
Check out our Facebook, Twitter, Instagram and LinkedIn pages
Follow us
Get in touch
Contact us to find out more about our department.
Department of Engineering
Durham University
Lower Mount Joy
South Road
Durham
DH1 3LE
Tel: +44 (0) 191 334 1700
Questions about studying here?
Check out our list of FAQs or submit an enquiry form.
Your Durham prospectus
Order your personalised prospectus and College guide here.